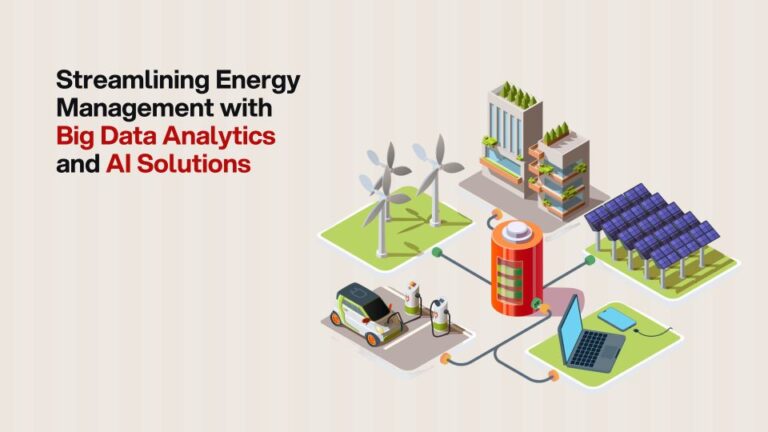
Contents
In the energy sector, managing resources efficiently is more important than ever. With increasing energy demands and the need for sustainability, businesses are turning to Big Data Analytics and Artificial Intelligence (AI) to solve complex challenges. These technologies are not just tools; they are strategies that transform how energy is monitored, distributed, and consumed.
What is Big Data Analytics?
Finding patterns and trends in vast amounts of data through collection, analysis, and interpretation is known as big data analytics. Among the many advantages that big data in the energy sector may offer are increased customer satisfaction, cost reduction, operational efficiency, and energy production optimization.
Big Data In Energy
Gathering appropriate data from various sources, such as sensors or meters placed on electricity lines or at client sites, marks the first step in big data analytics. Once collected, analysts use sophisticated algorithms to examine the data, uncovering patterns and correlations. For instance, a utility company can apply Big Data analytics to study historical usage data and weather forecasts, allowing it to predict future electricity consumption more accurately.
As a result, businesses gain valuable insights that help them make well-informed decisions about their operations. They can proactively invest in new technology or adjust pricing structures based on anticipated demand levels. This approach not only meets consumer needs but also minimizes environmental impact.
Furthermore, by continuously monitoring real-time consumption trends across their customer base, businesses can swiftly detect outages or other issues before they escalate into major problems.
The Big Data Analytics Market in Energy is set to experience significant growth. Experts project it will expand from USD 10.62 billion in 2025 to USD 17.95 billion by 2030, reflecting a CAGR of 11.07% during the forecast period (2025-2030)1.
Big Data Applications in Energy and Utilities
Energy systems generate an enormous amount of data every second from smart meters, IoT devices, energy grids, and renewable energy installations. The challenge is extracting meaningful insights from this data to optimize energy use and reduce costs. Big Data Analytics enables energy companies to:
- Monitor Energy Consumption: Advanced algorithms analyze consumption trends to help businesses identify inefficiencies and reduce waste.
- Forecast Energy Demand: Predictive models built on machine learning anticipate energy demand spikes, helping to stabilize supply and optimize resource allocation.
- Detect Anomalies: AI algorithms detect unusual patterns in energy use or system operations, flagging potential faults before they escalate.
- Improve Grid Reliability: Data from sensors and smart grids ensures smooth distribution of energy, even during peak times.
These capabilities help energy companies meet both operational efficiency goals and sustainability targets.
As per Mordor Intelligence, a leading market research firm based in India, the energy data analytics market is led by major tech companies like IBM, Microsoft, SAP, Dell Technologies, Intel, Accenture, Infosys, and Palantir Technologies. These leaders drive innovation through investments in AI, machine learning, advanced analytics, and cloud solutions tailored to the energy sector.
Types of Big Data Analytics
Big Data Analytics is categorized into four main types, each serving a specific purpose in extracting insights from data.
- Descriptive Analytics: Provides insights into what has happened in the past. It helps energy companies monitor historical energy consumption patterns or track grid performance.
Example: A dashboard displaying energy usage trends over the past year. - Diagnostic Analytics: Focuses on understanding why something happened by analyzing historical data. Useful when identifying the reasons behind a sudden increase in energy consumption or equipment failures.Example: Root cause analysis of power outages using sensor data.
- Predictive Analytics: Uses historical data and machine learning to predict future outcomes and forecast energy demand or predict equipment maintenance needs. Example: AI models predicting peak energy usage times to prepare grid operations.
- Prescriptive Analytics: Suggests optimal actions to take based on data insights and predictions. It is used when recommending energy-efficient strategies or automating responses to grid issues. Example: An AI system advising energy providers to shift load distribution during peak times to prevent overloads.
Find Your Perfect Software Outsourcing Partner
Unlock a world of trusted software outsourcing companies and elevate your business operations seamlessly.
Discover CompaniesTechnology Stack Behind Big Data Analytics and AI Solutions
To implement Big Data Analytics and AI in energy management, software companies rely on a robust tech stack and specialized tools. Below is a table summarizing some common technologies:
Category | Tool/Technology | Purpose |
---|---|---|
Data Storage | Hadoop, Amazon S3, Google BigQuery | Handle large-scale data storage and processing |
Data Processing | Apache Spark, Apache Flink | Real-time or batch processing of energy data |
Machine Learning Platforms | TensorFlow, PyTorch, Scikit-learn | Build and deploy AI and machine learning models |
Data Visualization | Tableau, Power BI, D3.js | Create visual insights for decision-making |
IoT Data Integration | MQTT, Kafka, Azure IoT Hub | Connect and process IoT data streams |
With these tools, energy companies can efficiently handle terabytes of data and transform them into actionable insights.
Want To Develop Big Data Analytics & AI Solutions?
For many energy companies, developing Big Data Analytics and AI solutions in-house can be time-consuming and expensive. This is why outsourcing to developing countries like India, Bangladesh, Pakistan, Vietnam, and Poland has become an attractive option.
- Cost Efficiency: Outsourcing reduces costs while maintaining high-quality outcomes.
- Access to Expertise: Countries like India, Bangladesh, Pakistan are home to skilled engineers proficient in data engineering, machine learning, and AI technologies.
- Scalability: Outsourcing teams can quickly adapt to changing project requirements and deliver results faster.
Outsourcing this expertise to trusted partners in developing countries allows energy companies to gain cutting-edge solutions while remaining cost-efficient.
Conclusion
Big Data analytics in the energy and utilities sector brings valuable advantages, such as boosting operational efficiency and providing real-time insights into customer behavior. It helps companies stay ahead of market trends and plan resources more effectively by predicting future demand. With clearer visibility into operations, providers can quickly spot issues and reduce risks related to safety and environmental compliance.
If you are exploring how Big Data Analytics and AI can help streamline your energy operations, contact Enosis Outsourcing today! Partner with top software outsourcing firms from our curated list of companies to get started on your cost-saving journey today.
References
[1] Big Data Analytics in Energy Sector Size & Share Analysis – Growth Trends & Forecasts (2025 – 2030) ↩