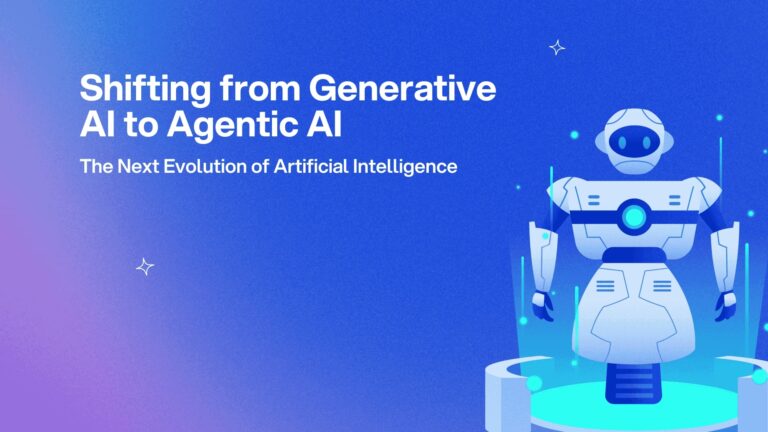
Contents
The transition from Generative AI to Agent AI signifies a substantial progression in artificial intelligence, with a focus on constructing more dynamic, adaptable, and context-aware systems. Shifting from Generative AI to Agentic AI leverages the capabilities of large language models (LLMs) to develop artificial agents that can manage intricate tasks and acclimate to evolving environments, making AI more autonomous and responsive. The transition from Generative AI to Agent AI signifies a substantial progression in artificial intelligence, with a focus on constructing more dynamic, adaptable, and context-aware systems. This advancement utilizes the capabilities of large language models (LLMs) to develop artificial agents that can manage intricate tasks and acclimate to evolving environments.
The Key Differences Between Generative And Agentic AI
Artificial intelligence (AI) has become the buzzword of our time. The term “Artificial Intelligence” frequently evokes images of robots and self-learning machines; however, AI is a broad field encompassing numerous distinct subfields. Two of the most talked-about developments today are generative AI and agentic AI. The key takeaway is that they operate differently. Understanding these differences is crucial to comprehending how AI is and will continue to transform our world.
Generative AI and agentic AI are primarily differentiated by their functions. Generative AI creates new content, while agentic AI focuses on achieving specific goals. Simply put, one creates, and the other acts.
Generative AI is largely static. Its output is based on the data it has learned; it does not adapt in real time or interact dynamically with the world, and operates within predefined boundaries. In contrast, agentic AI is dynamic. It constantly processes new information, learns from its environment, and adjusts its actions accordingly.
A key distinction also lies in the complexity of their objectives. While Generative AI typically operates on tasks that are narrow and well-defined, such as generating a paragraph of text or a digital painting, Agentic AI frequently addresses broader, multi-step goals that necessitate continuous decision-making and adaptation.
Despite their differences, generative AI and agentic AI aren’t mutually exclusive. Generative AI and agentic AI frequently collaborate effectively. For example, an agentic AI system might leverage generative AI to enhance communication or produce personalized content instantly. Envision a virtual customer service agent. This agentic AI manages the real-time interaction, making decisions based on user input and directing the conversation. Simultaneously, a generative AI component could develop nuanced and personalized responses to specific inquiries.
This synergy is also evident in areas such as robotics. For instance, a robotic chef could be equipped with both generative and agentic AI. The generative AI could create new recipes based on user preferences and available ingredients, while the agentic AI would manage the actual cooking process, ensuring that everything is prepared perfectly.1
Find Your Perfect Software Outsourcing Partner
Unlock a world of trusted software outsourcing companies and elevate your business operations seamlessly.
Discover CompaniesArtificial intelligence and Agents
Artificial Intelligence (AI) with the audacious goal of creating artificially intelligent systems comparable to or exceeding the intelligence of humans. By the early 1990s, many of the foundational AI algorithms in use today, including Neural Networks, Reinforcement Learning, Symbolic Reasoning, and Evolutionary Algorithms, had already been discovered and were achieving impressive results in constrained and straightforward problem domains. The remaining challenges appeared to be generality and scale.2
Integrating these algorithms, each with potential in a specific aspect of human intelligence, into a single coherent system was challenging. Additionally, scaling them to address a variety of real-world problems for which they were not trained proved unattainable. Consequently, the field of AI shifted its focus towards agent-based systems.
Artificial intelligence agents can be considered human representatives, navigating the internet and completing tasks on our behalf. The logical progression of this type of framing was to consider the outcome of two agents encountering each other. Comprehensive frameworks and interagent languages were developed to address this. Another interesting avenue for exploration was systems composed of multiple agents, configured to work together to accomplish tasks and achieve goals.
Breaking a problem into smaller parts, solving each, and combining the results may solve the larger problem. Some multiagent approaches use this strategy. A bonus of such systems was the fact that they are robust and extensible. Adding or removing specialized agents from a group would introduce or remove functionality without having to re-engineer the entire system.
The lines between generative and agentic AI will blur further as these two types of AIs evolve. Advances in technology could lead to systems that seamlessly integrate creation and action, leveraging the capabilities of both functions. Imagine an AI that not only generates innovative ideas but also implements them autonomously—a paradigm shift for industries spanning healthcare to manufacturing.
Conclusion
The transition from Generative AI to Agentic AI represents a pivotal shift in artificial intelligence, progressing from static content generation to dynamic, goal-driven adaptability. Shifting from Generative AI to Agentic AI moves beyond merely producing text, images, and other media to actively engaging with the environment, making decisions, and executing tasks. While Generative AI excels in content creation, Agentic AI enhances these capabilities by enabling systems to act independently. These two AI models are not mutually exclusive but rather complementary, with the potential for seamless integration. As AI technology advances, the fusion of generative and agentic capabilities will redefine automation, enabling intelligent systems that not only create but also act, innovate, and adapt autonomously. This evolution paves the way for AI-driven transformations across various sectors, from customer service and robotics to healthcare and beyond.
References
[1] Generative AI Vs. Agentic AI: The Key Differences Everyone Needs To Know ↩
[2] AI and agents ↩